Getting to Grips with AI!
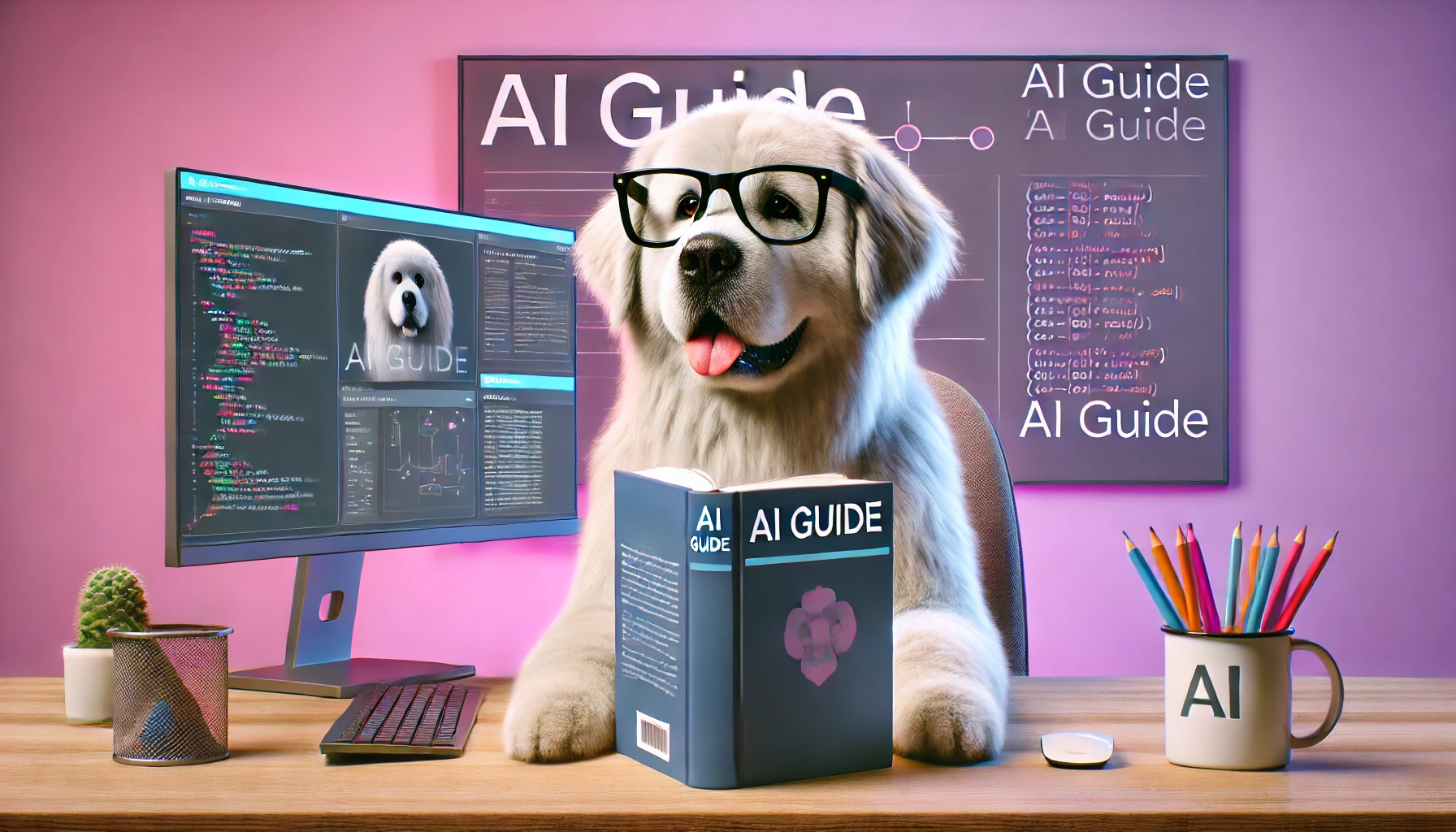
Understanding the myriad of terms used to talk about AI can be challenging, but we're here to help!
Artificial intelligence (AI) is transforming the tech landscape, with companies worldwide integrating AI to drive innovation and efficiency. However, the field is rife with jargon and complex concepts, making it challenging to grasp for those not deeply embedded in tech.
At Yopla, our mission is to make business better by aligning people and technology. To help you navigate the AI landscape, we’ve compiled a comprehensive guide to some of the most common AI terms and concepts, explaining what they mean and why they matter.
What Exactly is AI?
Artificial intelligence (AI) refers to the development of computer systems that can perform tasks typically requiring human intelligence. This includes learning, reasoning, problem-solving, and understanding natural language.
AI is a broad field encompassing various technologies and methodologies, often used interchangeably with machine learning, deep learning, and neural networks.
“Artificial intelligence will have a more profound impact on humanity than fire, electricity and the internet.” - Sundar Pichai, CEO of Google
Key Terms in AI
Machine Learning
Machine learning (ML) is a subset of AI where algorithms are trained on data to make predictions or decisions without being explicitly programmed for the task. ML systems improve over time as they are exposed to more data.
Artificial General Intelligence (AGI)
AGI refers to a type of AI that possesses the ability to understand, learn, and apply knowledge across a wide range of tasks at a level comparable to a human. While current AI systems are specialised, AGI aims to be versatile and adaptable.
Companies like OpenAI are investing heavily in AGI, which holds great promise but also raises ethical and safety concerns.
Generative AI
Generative AI is a type of AI that can create new content, such as text, images, music, and code. Examples include OpenAI's GPT models and Google's Gemini.
These systems are trained on large datasets and can generate outputs based on the patterns they have learned.
Hallucinations
In AI, hallucinations refer to instances where generative models produce confident but incorrect or nonsensical answers. This happens because the models generate responses based on their training data, which might not cover every possible scenario accurately.
Bias
Bias in AI occurs when the training data or algorithms lead to unfair or discriminatory outcomes. For example, facial recognition systems have been shown to have higher error rates for certain demographic groups. Addressing bias is crucial to ensure AI systems are fair and equitable.
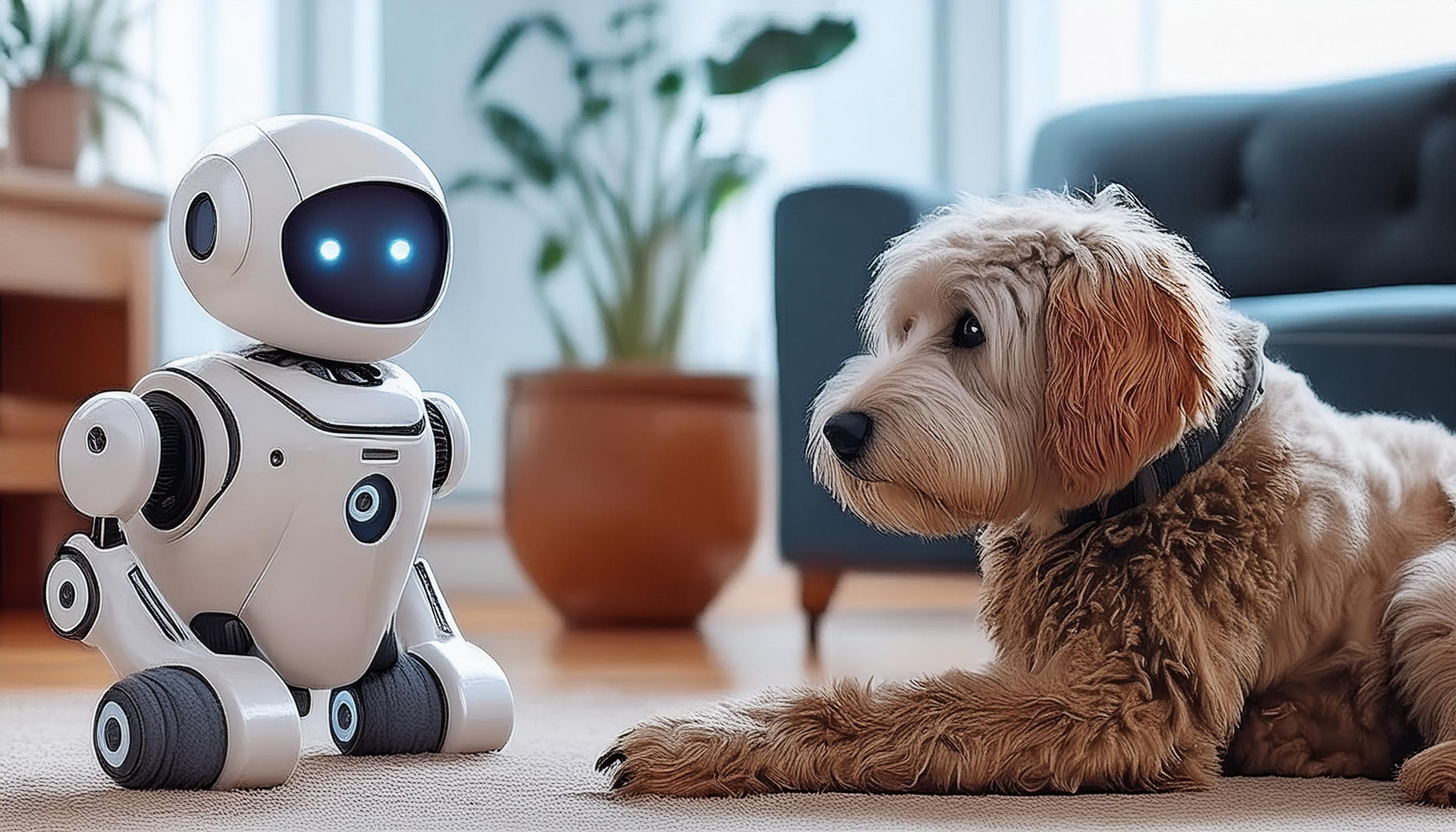
Understanding AI Models
AI Model
An AI model is a mathematical framework designed to solve specific problems or perform tasks by learning from data. Models can range from simple linear regressions to complex neural networks.
Large Language Models (LLMs)
LLMs, such as OpenAI’s GPT-4 and Anthropic’s Claude, are a type of AI model trained on extensive text data to understand and generate human language. They can perform tasks such as translation, summarisation, and question-answering.
Diffusion Models
Diffusion models are used to generate images from text prompts. They work by adding noise to images and then learning to reverse this process to create clear images. This technology is also applied to audio and video generation.
Foundation Models
Foundation models are large-scale AI models trained on diverse datasets, making them versatile for various applications. Examples include OpenAI’s GPT, Google’s Gemini, Meta’s Llama, and Anthropic’s Claude. These models can handle multiple data types and tasks without requiring task-specific training.
Frontier Models
Frontier models are the next generation of AI models under development. These models promise to be more powerful and capable than current models, potentially transforming industries and posing new challenges.
Training AI Models
“We need to be careful about the data we use to train AI systems. If the data is biased, the AI will be biased.” - Joy Buolamwini, Founder of the Algorithmic Justice League
Training AI models involves teaching them to recognise patterns and make predictions by processing large datasets. This requires significant computational resources and advanced hardware such as GPUs.
The process includes the following components:
Training Data
The data used to train AI models, which can include text, images, audio, and video. The quality and diversity of training data are crucial for the model's performance.
Parameters
Parameters are the variables within an AI model that are adjusted during training to improve accuracy. The number of parameters can indicate the model's complexity and capacity.
Natural Language Processing (NLP)
NLP is a field of AI focused on enabling machines to understand and generate human language. Applications include chatbots, voice assistants, and language translation tools.
Inference
Inference is the process of using a trained AI model to make predictions or generate outputs. This is what happens when you interact with AI applications like chatbots or image generators.
Tokens
Tokens are units of text (words, subwords, or characters) that AI models process. The model's ability to handle more tokens can improve its performance in understanding and generating text.
Neural Networks
Neural networks are a type of AI architecture inspired by the human brain, consisting of interconnected nodes (neurons) that process data. They are fundamental to many AI systems, especially in deep learning.
Transformer
Transformers are a neural network architecture that has revolutionised NLP by enabling models to handle long-range dependencies in text. They use an attention mechanism to process sequences of data efficiently.
Retrieval-Augmented Generation (RAG)
RAG combines the generation capabilities of AI models with external data retrieval to improve accuracy. It allows models to access information beyond their training data, reducing hallucinations and enhancing reliability.

AI Hardware
AI systems require robust hardware to process large datasets and perform complex computations. Key components include:
Nvidia’s H100 Chip
A leading GPU for AI training, known for its efficiency and performance in handling AI workloads.
Neural Processing Units (NPUs)
Specialised processors designed for AI tasks, providing faster and more efficient performance than general-purpose CPUs or GPUs.
TOPS (Trillion Operations Per Second)
A measure of a chip's capability in executing AI operations, often used to highlight the performance of AI hardware.
Leading AI Companies and Tools
Several companies are at the forefront of AI development, each contributing unique tools and innovations:
OpenAI / ChatGPT
Known for its popular AI chatbot, ChatGPT, which has brought generative AI into the mainstream.
Microsoft / Copilot
Microsoft integrates AI into its products through Copilot, which is in some applications an extension of OpenAI's GPT foundations.
Google / Gemini
Google’s AI models power various products, from search enhancements to smart assistants.
Meta / Llama
Meta’s open-source AI model, Llama, aims to democratise AI research and development.
Apple / Apple Intelligence
Apple incorporates AI features into its ecosystem under Apple Intelligence, enhancing user interactions with devices.
Anthropic / Claude
Founded by former OpenAI employees, Anthropic focuses on creating AI models with a strong emphasis on safety and ethics.
xAI / Grok
Elon Musk’s AI venture, Grok, aims to push the boundaries of AI capabilities.
Hugging Face
A platform that provides a directory of AI models and datasets, fostering collaboration in the AI community.
How Yopla Can Help
Understanding the multitude of terms and concepts in the field of AI can feel overwhelming. The rapid pace of technological advancement means new terminologies and methodologies are always emerging, making it hard for anyone not deeply involved in tech to keep up. From machine learning and neural networks to generative AI and large language models, the sheer volume of information can be daunting! Plus, the subtle differences between similar concepts and the details of how these technologies are used in real-world scenarios can add to the confusion.
At Yopla, we get it! That's why we're here to help you make sense of it all.
Our mission is to make AI accessible and understandable for businesses of all sizes. We're dedicated to providing clear, practical insights that help you navigate the AI landscape with confidence. We’ll work closely with you to find the AI solutions that best meet your organisation's unique needs, making sure you can leverage AI to drive innovation and efficiency.
With Yopla by your side, you'll have a trusted partner to simplify the complexity of AI and deliver real benefits for your business.
For more information, contact us today on team@yopla.co.uk, or book a meeting now.